Ten steps to ethics-based governance of AI in healthcare
AI’s ability to interpret data relies on processes that are not transparent, making it difficult to verify and trust outputs from AI systems.
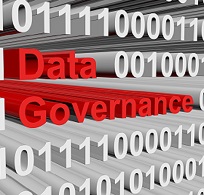
data-governance-three.jpg
More for you
See AllLoading data for hdm_tax_topic #better-outcomes...